We recently broke down how to build AI agents using plan-and-execute loops. These artificial intelligence agents use the reasoning capabilities of large language models (LLMs) to autonomously make decisions on how to achieve their given task, from ordering pizza to retrieving account information.
But single AI agents have a ceiling on how complex of tasks they can reliably automate on their own. In those cases, businesses are better served by multiple intelligent agents cooperating together. These multi-agent AI systems allow us to solve problems of increasing complexity by delegating specialized tasks to specific agents.
Knowing when to expand from a single AI agent to multiple agents can be difficult, but eventually it becomes necessary. As we add more complexity to a single agent, our massive toolbox becomes more of a burden. Also, a single agent can quickly become difficult to debug and increase the possibility of our agent making mistakes.
But if we expand our workflows to include multiple specialized agents we can add performance, clarity, and adaptability to completing our given goal. Let’s step through a use case where we’ll highlight:
- Why single agents start to fail
- When to move to a multi-agent approach with an example
- What benefits and challenges come with multi-agent AI systems
We’ll start with what makes most single AI agents start to fail.
When Do Single AI Agents Start to Fail?
A single-agent approach can make sense at first (i.e., one AI agent that can do everything from navigating a browser to dealing with file operations). Over time though, as the tasks become more complex and the number of tools grow larger, our single-agent approach will start to be challenging.
We will notice effects when the agent starts to misbehave, which can result from:
- Too many tools: The agent gets confused on which tools to use and/or when.
- Too much context: The agent’s increasingly large context windows contain too many tools.
- Too many mistakes: The agent starts to produce suboptimal or incorrect results due to overly broad responsibilities.
When we start automating multiple distinct subtasks such as data extraction or report generation, it might be time to start separating responsibilities. By using multiple AI agents, where each agent focuses on its own domain and toolkits, we can enhance the clarity and quality of our solution. Not only does this allow the agent to become more effective, but it also eases the development of the agents themselves.
Example Use Case From Finance
Let’s walk through a common example in finance. Suppose we have an agentic workflow and data pipeline that takes in a user query, interacts with a database, and does analysis to generate a report.
1. Single-agent scenario
In the case we have a single agent, this agent is responsible for:
- Taking the user query to create a plan and execute it
- Querying and reading the database with financial data
- Loading the data into a pandas DataFrame for analysis
- Generating charts with matplotlib
- Creating a final report summarizing the insights
Our-do-it-all single agent manages file I/O, data analysis, visualization, and reporting. Each of those tasks is complex enough to warrant its own specialized agent.
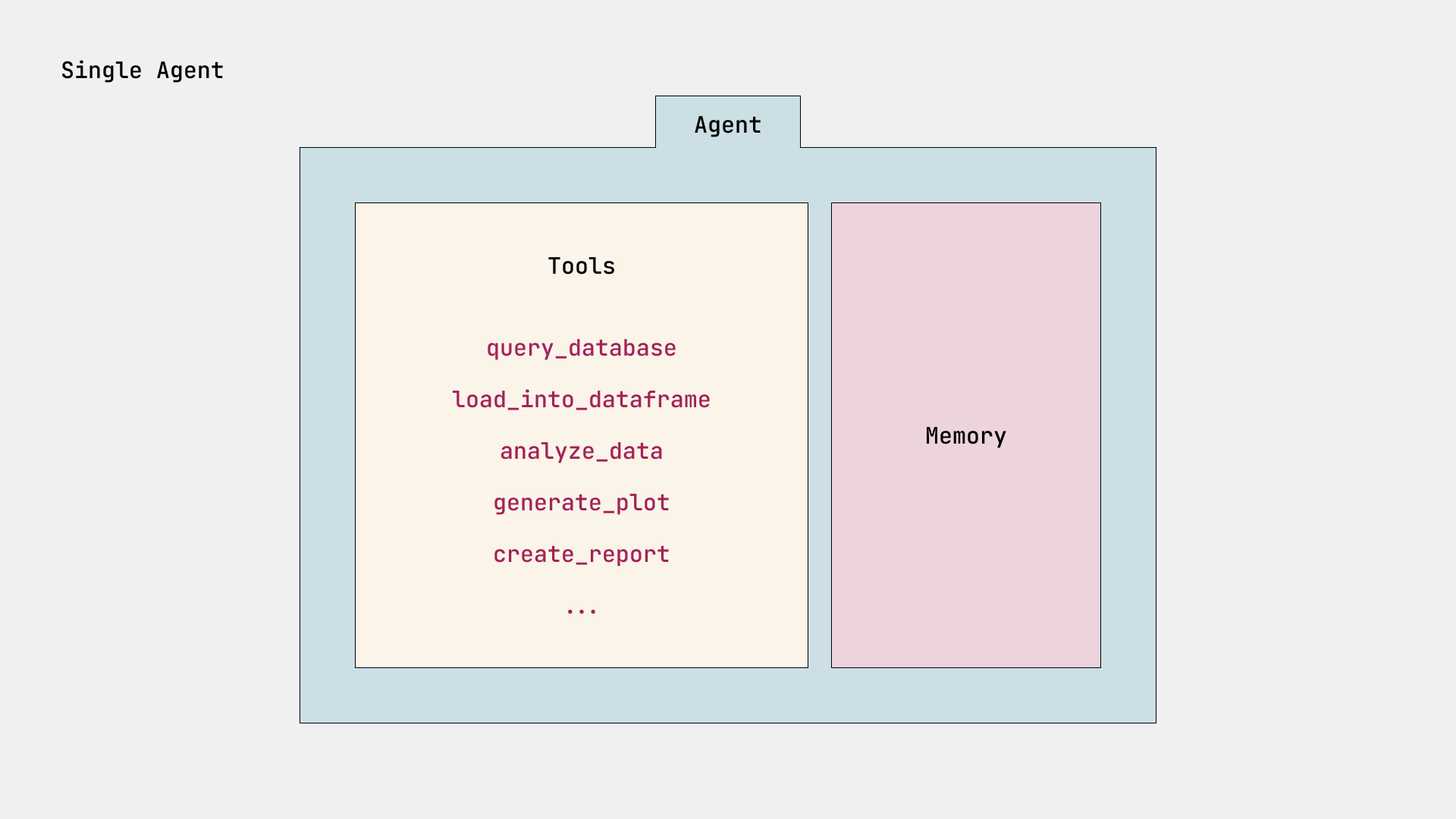
That’s a lot to track and will inevitably lead to more complex prompts and a higher chance of error, especially when adding more features and use cases.
2. Multi-agent scenario
If we instead use multiple agents, we can break down our workflow into manageable agents targeting specific tasks and responsibilities. There are a few approaches to a multi-agent setup. We could choose an orchestrator setup where one agent chooses other agents. Alternatively, we could have multiple agents optimize our process by choosing the next best agent based on the next steps of completing the goal.
We’ll take a look at the orchestrator method, which starts with our Orchestrator Agent:
- Orchestrator Agent: Decides which agent to call and in what order.
- Database Agent: Queries data from a database.
- Analysis Agent: Analyzes the returned data.
- Graph Agent: Generates visualizations based on analysis.
- Report Agent: Creates a report document to share the insights from analysis.
The Orchestrator Agent works similar to how a single agent chooses tools, except now it chooses which specialized agent to call, as shown in the graphic below.

The separation of responsibilities between these agents leads to clearer logic and a more scalable architecture. This allows us to logically separate out the specific scope and tools available to a specific agent.
Building Multi-Agent AI Systems in Python
To set up the orchestrator framework we explored above, we’ll need to specify several things in our code.
Core multi-agent components
We can expand our core components of agents to now include an orchestrator:
- Orchestrator Agent: The agent responsible for choosing which agents to use to complete a goal.
- Agents: Autonomous AI with access to memory and tools of a specified domain to complete a goal.
- Memory: Each agent can maintain its own memory or state.
- Tools: Functions that the agent has access to so it can complete its task.
For our simplified demo code, let’s consider an agentic workflow for data analytics that can fetch data, analyze it, generate a plot, and output a report.
Agentic flow
A user will provide a prompt such as, “Generate a quarterly report from the database for Q4 2024.”
The Orchestrator Agent will then decide how best to accomplish this task using the other agents. The plan might look like this:
- Database Agent:
query_database
tool to pull the Q4 2024 data. - Analysis Agent:
load_into_dataframe
andanalyze_data
tools to load and derive insights. - Graph Agent:
generate_plot
tool where visualizations can be created from insights. - Report Agent:
create_report
to combine insights and graphs into a final document.
Now let’s pull all of this together in our code.
Example code
Below is a brief example to illustrate multiple agents working together. We’ll start with a similar structure and patterns as our previous post on building single AI agents, but now use multiple agents and an orchestrator.
We’ll start with our imports and Base Agent class.
Above, we create a Base Agent that all of the other Agent Classes will extend. This will print what agent is running and the output.
Now we’ll build our Specialized Agents.
The database, analysis, graph, and report agents target their respective tasks. Having the specialized agents focused on specific outcomes, we can reduce the likelihood of errors as we add new features and update our agents.
From here, we’ll introduce the Orchestrator Agent.
The Orchestrator Agent is the heart of our process. Very similar to plan-and-execute, we use this pattern again to show at a high level how orchestration can happen, where one agent plans and executes other agents to solve a task.
Last, we arrive at our main execution, “Generate a quarterly report from the database for Q4 2024.”
Our main execution above will take in our user_prompt of “Generate a quarterly report from the database for Q4 2024.” and run our Orchestrator Agent. This will kick off our multiagent workflow.
Now, here’s the output from our example prompt.

To summarize, this code:
- Shows how the orchestrator can coordinate processes between agents, making the system easier to scale and modify
- Gives each agent a narrower set of responsibilities to reduce confusion and increase reliability
- Illustrates how multiple agents can work together to solve complex problems more effectively than single agents alone
When to Move From a Single Agent to Multiple Agents
Here are three key times you should consider expanding from a single agent to multiple agents.
1. Large number of tools with different scopes
If your agent handles file I/O, database queries, data analysis, and visualizations, it may be too broad. Splitting agents by specific domains helps simplify the context and reduce agent and developer confusion.
2. Performance and reliability issues
When a single agent becomes too large or complex, it may start to choose the wrong tools or fail tasks due to overly broad contexts.
3. Complexity
Having distinct agents keeps responsibilities focused and manageable. For example, a “DatabaseAgent” that only queries data is simpler to read and write (as well as more reliable) than a general-purpose single agent doing it all.
The Benefits of Expanding to Multiple Agents
Multi-agent systems offer AI practitioners lots of advantages over single-agent systems. Three benefits that stand out include:
- Increased capabilities: Dividing workloads between multiple agents allows AI to find solutions to increasing complex problems.
- Enhanced specialization: With each agent tuned to a specific domain, tasks become more focused and consistent.
- Scalability: Multi-agent AI systems can easily add new agents as the need arises, avoiding overloading a single agent.
Of course, some of these benefits bring added responsibilities that practitioners should be aware of.
Challenges of Multi-Agent Systems
As for the challenges of orchestrating multiple agents, the following are worth keeping top of mind:
- Cost: As more agents are involved and more LLM calls are created, the cost associated with completing the goal will increase.
- Debugging complexity: As multiple agents are incorporated, knowing where things are breaking can become difficult.
- Clear boundaries: As more tools are added to more agents, knowing when to increase or decrease one agent over the other can become muddied.
- Overhead: The developer is ultimately responsible for identifying where an agent would work best.
Solutions will likely emerge for these challenges as agentic AI matures. For instance, Anthropic recently announced Model Context Protocol (MCP), the first attempt at an industry standard for how AI systems and processes integrate with each other. An open source standard like that could positively impact each of the issues mentioned above if developers follow the recommended agent patterns.
Build the Multi-Agent Systems Your Business Needs
Moving from a single-agent to multi-agent architecture can improve the results and scalability of your application, especially as it grows in complexity. By separating responsibilities to dedicated agents, you can carry out more sophisticated tasks and enhance the capabilities within your workflows.
As the field evolves and agentic frameworks mature, we’ll see even more advanced patterns to handle large-scale multi-agent systems. With careful planning, multiple agents can empower your systems to take on bigger challenges with greater reliability and efficiency.
If you need help developing your agentic AI systems, we can help out. Our experience spans AI strategy governance, generative AI experiences, and voice. Learn more about our Data & AI Consulting services.